Home • Blog • Multi-armed bandit vs A/B test: choose your fighter
Multi-armed bandit vs A/B test: choose your fighter
Table of Contents
ToggleIf you’re stuck on choosing a color for CTA buttons, you’re not the only one. Honestly, we don’t know either. Good thing A/B testing knows.
A/B testing or split testing – the most common method of conversion optimization. Here’s how it works:
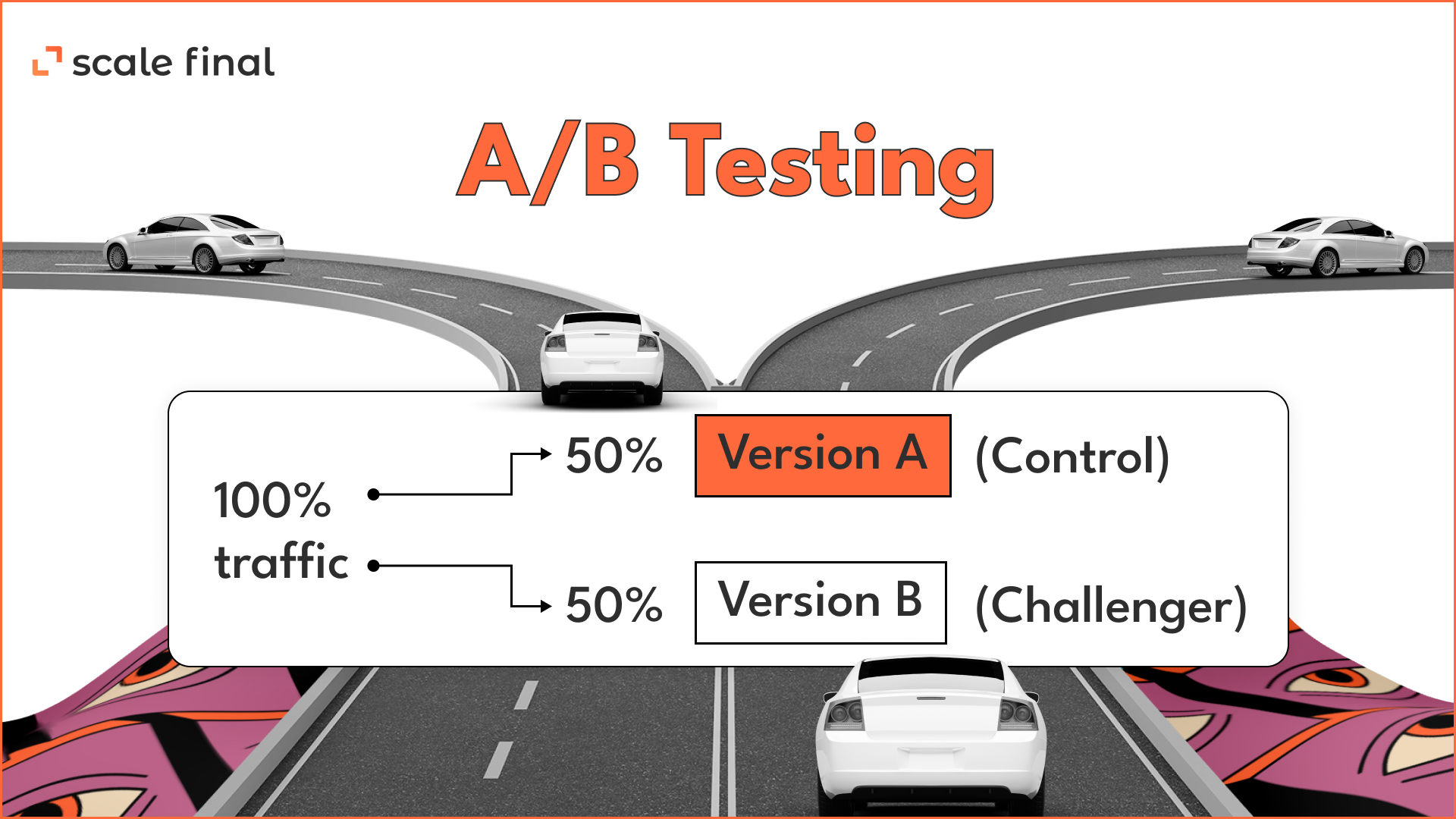
Traffic is distributed among 2 versions of your digital property. 50% of the traffic goes to the control version and 50% to the challenger version. This continues until the there’s enough data to decide which version is more efficient.
Two problems stand out in the application of this method:
- Testing abruptly shifts from research to practice, whereas a smoother transition would be more appropriate.
- In the data collection phase, resources are aimlessly wasted on testing the worst option in order to collect as much data as possible.
Missed a detailed explanation of the A/B testing? Learn more in CRO From Scratch. Part 2.
For we’re about to change the percpective now, and tell you about the multi-armed bandit testing.
The difference between the multi-armed bandit algorithm and A/B testing is how the experiment is split between the exploration phase (getting enough results to make a decision) and the exploit phase (redistributing traffic).
In split-testing, traffic is studied first and then redistributed to the only effective version:
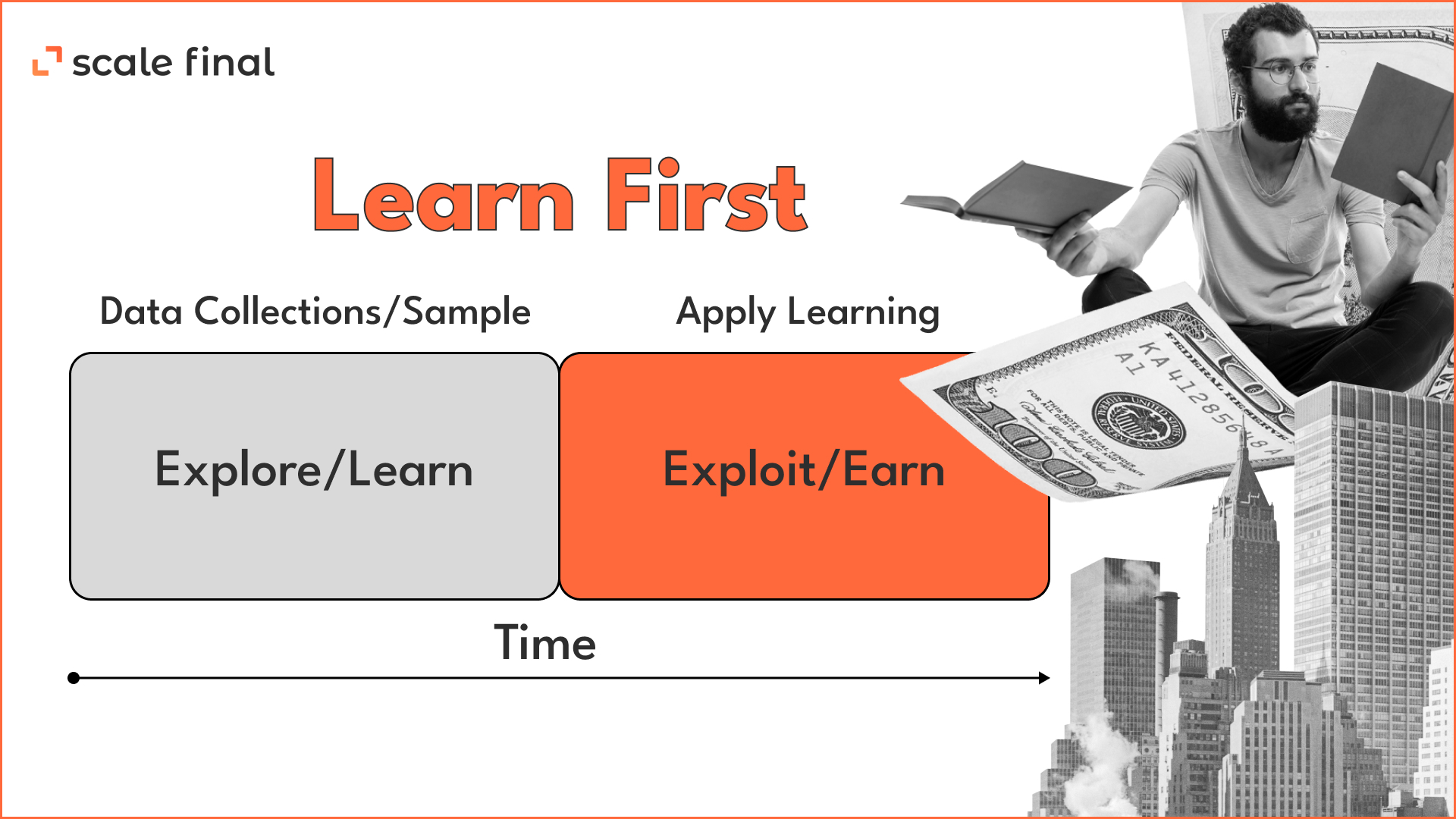
Multi-arm bandit solves the resource allocation dilemma between explore and exploit phases in a different way. Instead of two separate periods, it runs fluidly. Bandit testing is adaptive and allows you to simultaneously study and dynamically allocate traffic based on the data obtained:
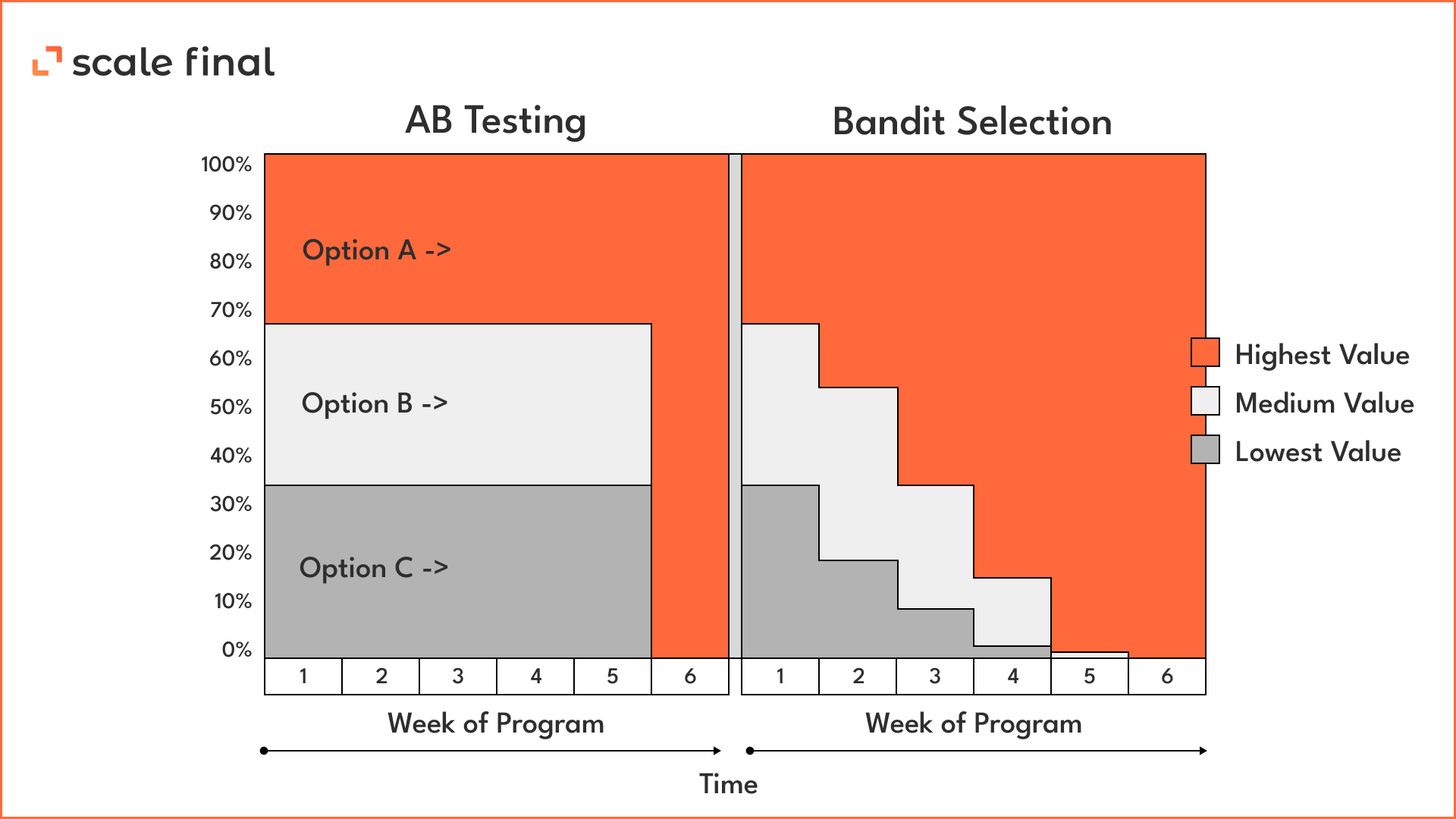
So bandit testing minimizes the cost and the difference between what you really get and what you could have gotten if you had chosen the best option at each stage.
Why the multi-armed bandit test minimizes costs?
Running A/B tests for different versions of your digital property is expensive. You need to buy traffic for Apple Search Ads, Facebook Ads, and Google Ads to get a sufficient number of users for reliable results.
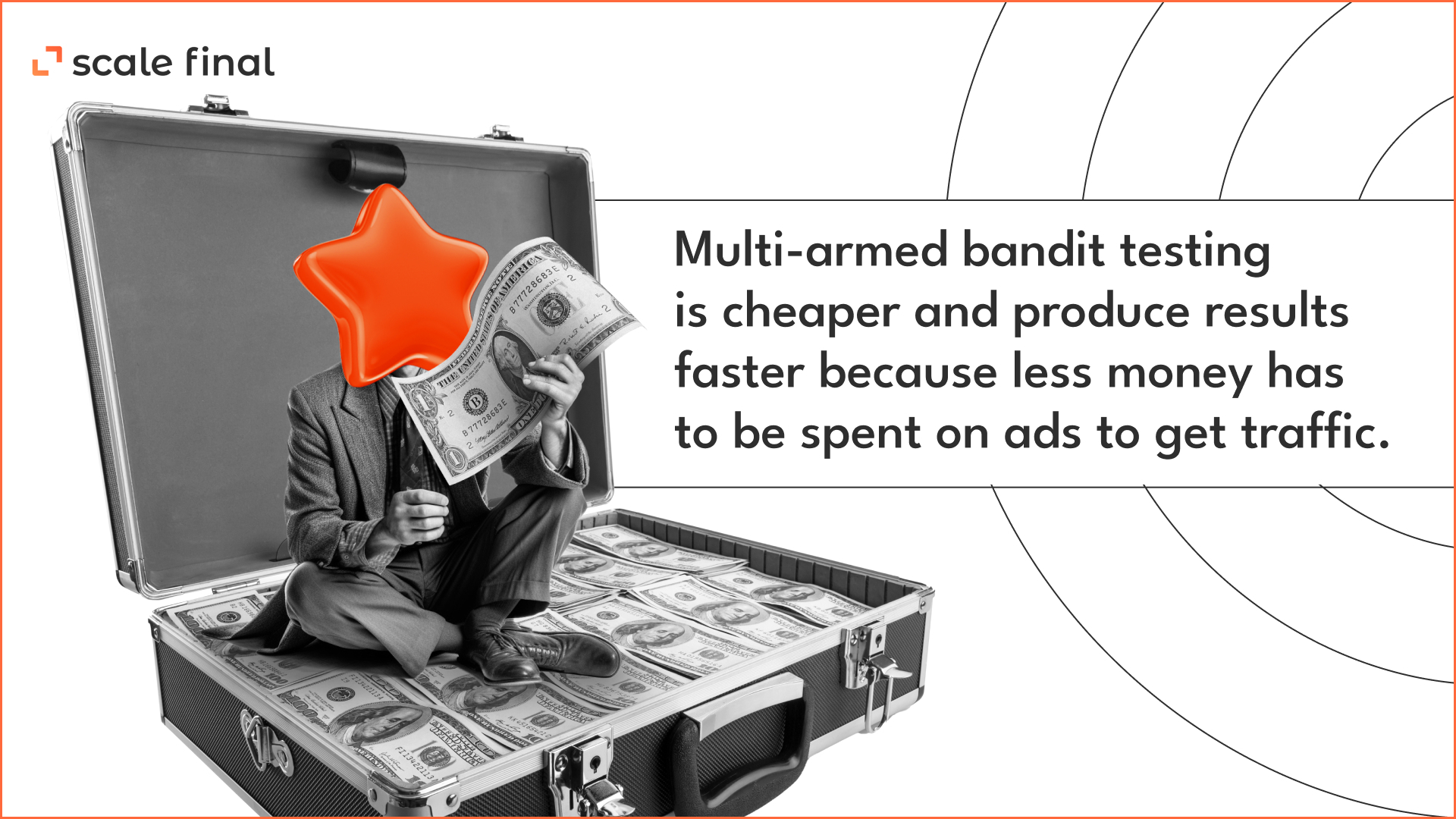
Of course, sometimes cutting corners is not an option. If you make poor decisions in the design or pre-launch phase of your product development, it can turn into a disaster.
However, some things require quick results and you can tolerate uncertainty. For example, running experiments before you launch a seasonal promotion or limited-time event.
When to use multi-armed bandits?
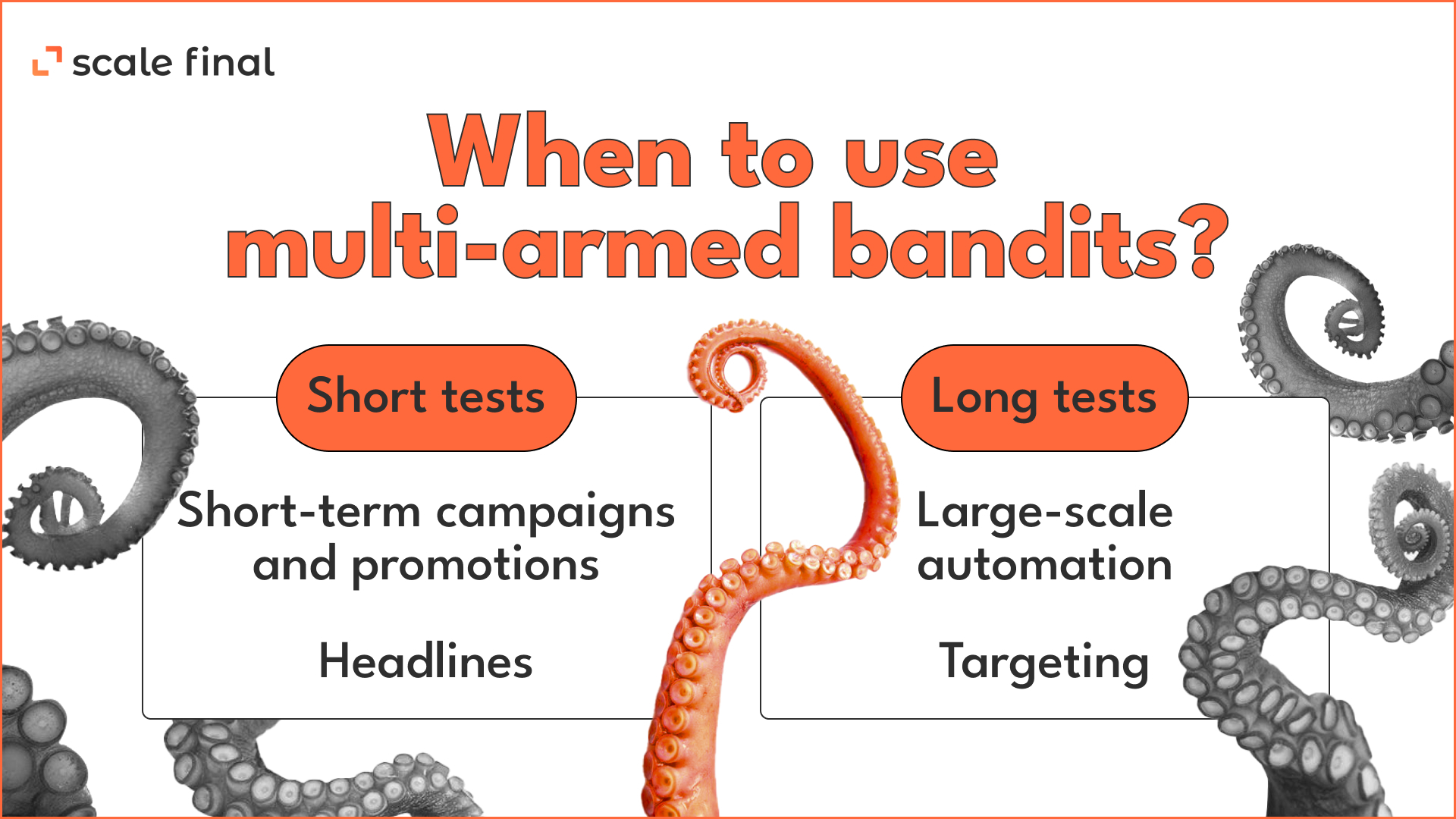
Bandit algorithms are suitable for short-term testing for obvious reasons. If A/B tests were used, there would be no time to apply the data obtained. Bandits allow you to change the course of the tests based on the results in real time. I.e. traffic directs to the best version faster. We recommend to use them when time resources are limited for both explore and exploit phases.
Short-term testing
Headlines
Headlines are the best objects for bandit algorithms. Why apply the classic split test to headlines if, by the time you figure out which option is best, the actual time for that headline has already passed? News has a short life cycle, and bandit algorithms will quickly figure out which headline is more effective.
Short-term campaigns and promotions
Similar to headlines, you lose a lot if you prefer A/B testing and your campaign only runs for a week. You obviously don’t want to experiment with half your traffic – by the time you get reliable results, the campaign will expire, and there won’t be time to use the successful options.
Long-term testing
Surprisingly, bandit tests are also effective in the long term and for continuous testing.
Set it and forget it (large-scale automation)
Since bandit algorithms redirect traffic to more efficient versions, you get a minimal-risk solution for long-term optimization. If you’re wondering how to decide in which order to display screenshots on your app’s product page, a multi-armed bandit test can make things very simple. Just automate the task and let it work.
Targeting
Another example is targeting, which is optimal for displaying specific content and ads in custom apps. The contextual bandit algorithm helps you avoid the typical mistakes with audience heterogeneity. Bandit algorithm solves this problem by applying the targeting rules derived from research to a general segment of users, while continuing to look for different rules for less common users.
Conclusion
Overall, multi-armed bandit testing can be a powerful tool for marketers to optimize their strategies and improve their ROI, but it requires careful planning and execution to be effective.
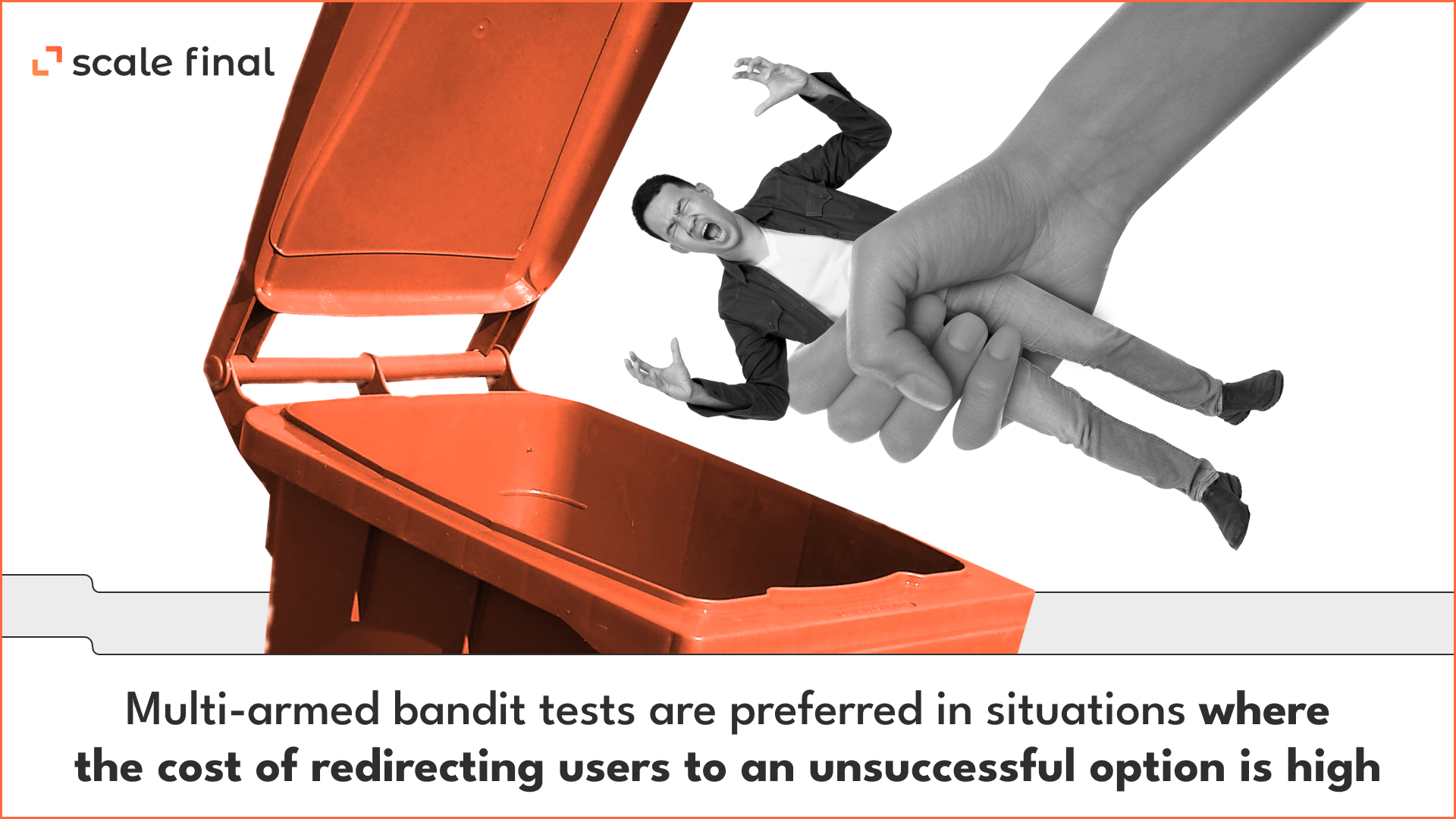
For example, multi-arm bandit tests are more efficient for early-stage startups with insufficient user traffic because they require a smaller sample size and provide faster results than A/B tests.
In addition, bandit algorithms can quickly determine the winner when testing multiple variants. Unlike A/B testing, which can only test two groups at a time, multi-armed bandit testing can test four to eight variants simultaneously.
In some cases, A/B testing is still preferable, such as when testing a new feature or design where less information is available in advance about how users will respond.
Ultimately, the decision between multi-arm bandit testing and A/B testing depends on the specific goals and constraints of the marketing campaign, as well as available resources and expertise.
Author
-
Product Manager
I'm equal parts strategic thinker and creative doer, with over 6 years of experience in digital marketing. I started out as a creative copywriter, and now I'm all in product management. Fun, inspiring and slightly caffeinated.
View all posts