Home • Blog • The evolution of Google Search Engine
The evolution of Google Search Engine
Table of Contents
ToggleBeing found on the Internet isn’t just a luxury. It’s a necessity. As you grapple with the ever-changing digital landscape, it’s crucial to understand how Google search has evolved over the years and what that means for modern search engine optimization.
Take a journey from its keyword-based beginnings to today’s machine learning algorithms.
From keywords to user intent
In the 90s, search engines ranked pages based on keywords. You just needed to include your keywords as much as possible, and you’d get a good ranking.
And then came Google. The IT giant was founded in 1998 and introduced its PageRank algorithm based on inbound links. This revolutionized SEO and made Google the top search engine.
2003 the first major Google evolution update was birthed – the Florida update. It was designed to combat link spammers. Google began using statistical link analysis to find natural data points (anchors, link patterns etc.) Google still uses this approach. That’s why statistically natural data points are essential even today for SEO.
However, staying under the radar was relatively easy: relying on keyword density, EMDs, and spam links with XRumer.
In 2005, Jasper and BigDaddy updates followed, and then there was a break until the Panda update (2011 – it eliminated content farms of the 00s) and the Penguin update (2012 – exact anchors started being potentially detrimental).
Things were beginning to change for link spammers.
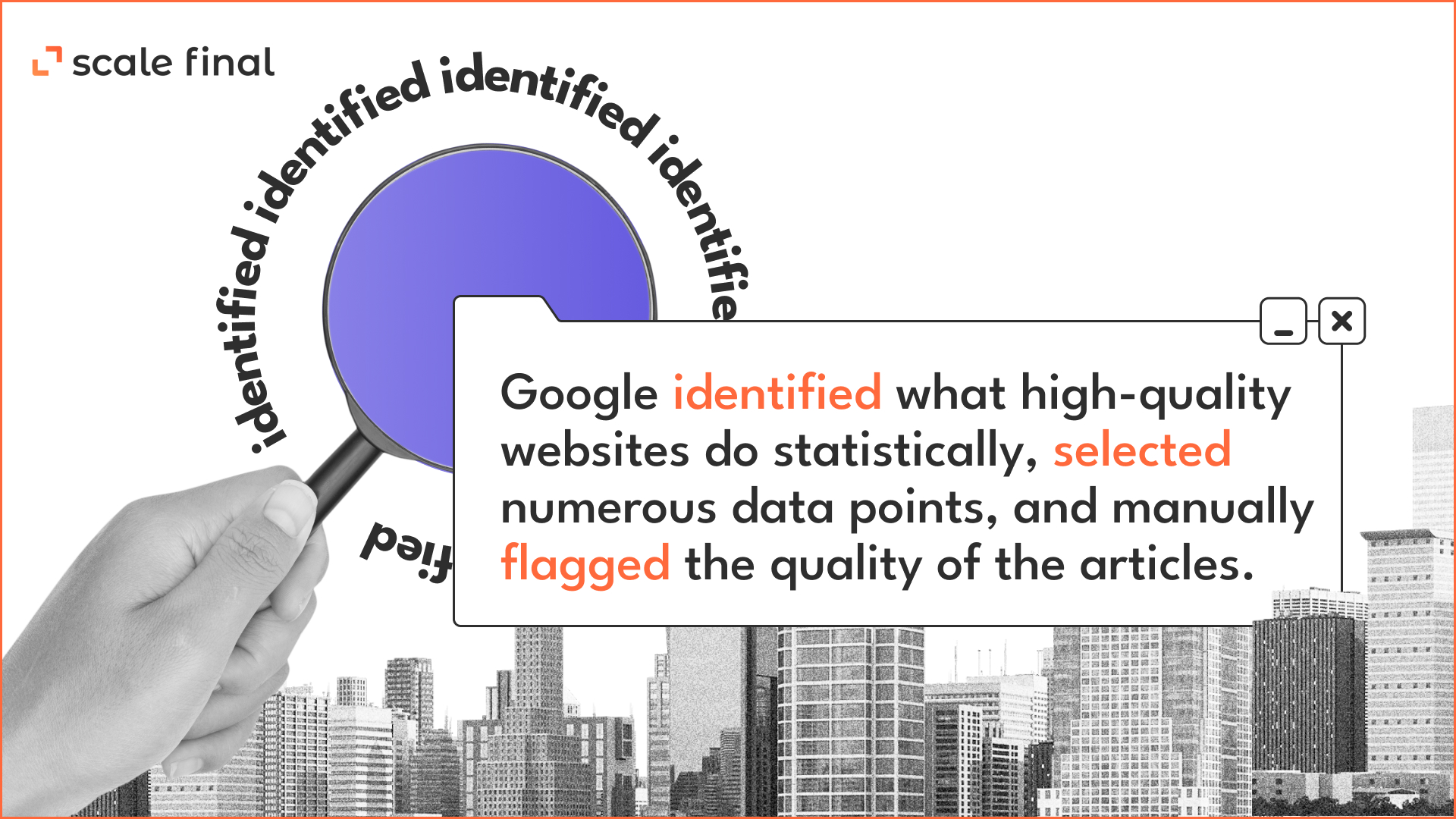
The following year, the Hummingbird update happened. Google tried to have the user’s intent behind the search in its computer mind. Instead of searching for sites based on user query as it was typed, it looked for those sites that could match a user’s intent.
This brought it much closer to modern SEO.
The rise of machine learning in Google Search
RankBrain update was released in 2015 and brought with it machine learning. AI relies on a Knowledge Graph filled with entities (currently, 8 billion of them) and facts (800 billion).
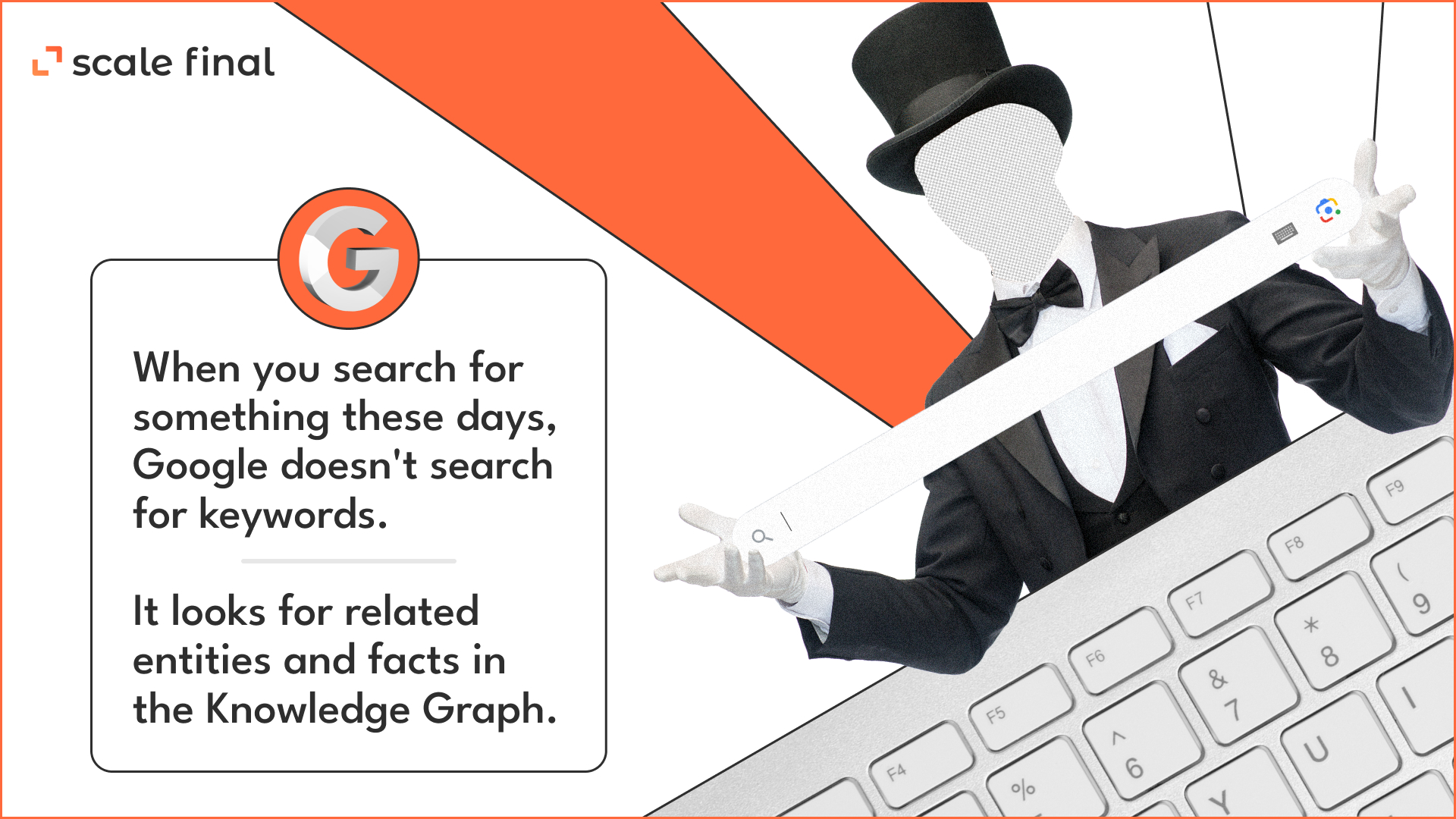
It’s contextual-based, so it can tell the difference between searching for an apple (fruit) and Apple (the tech company). I
t can recognize that the user is searching for Paris no matter if you type “the capital of France” or “What is the capital of France”.
To further understand user intent, Google keeps track of a search chain. It can predict with some accuracy what search will follow the previous one.
Furthermore, machine learning generates questions to fill in the missing information. It searches the documents indexed on the net and then updates the Knowledge Graph with answers. That’s why PAA sites dominated the net, consisting purely of Q&A until Google stopped it with further updates.
In 2020, Google rolled out the Bert core update. It further improved AI’s understanding of user intent and the ability to find entities, generate and answer questions. It also brought natural language processing to understand search queries semantically better.
So the current authority came into play with full force.
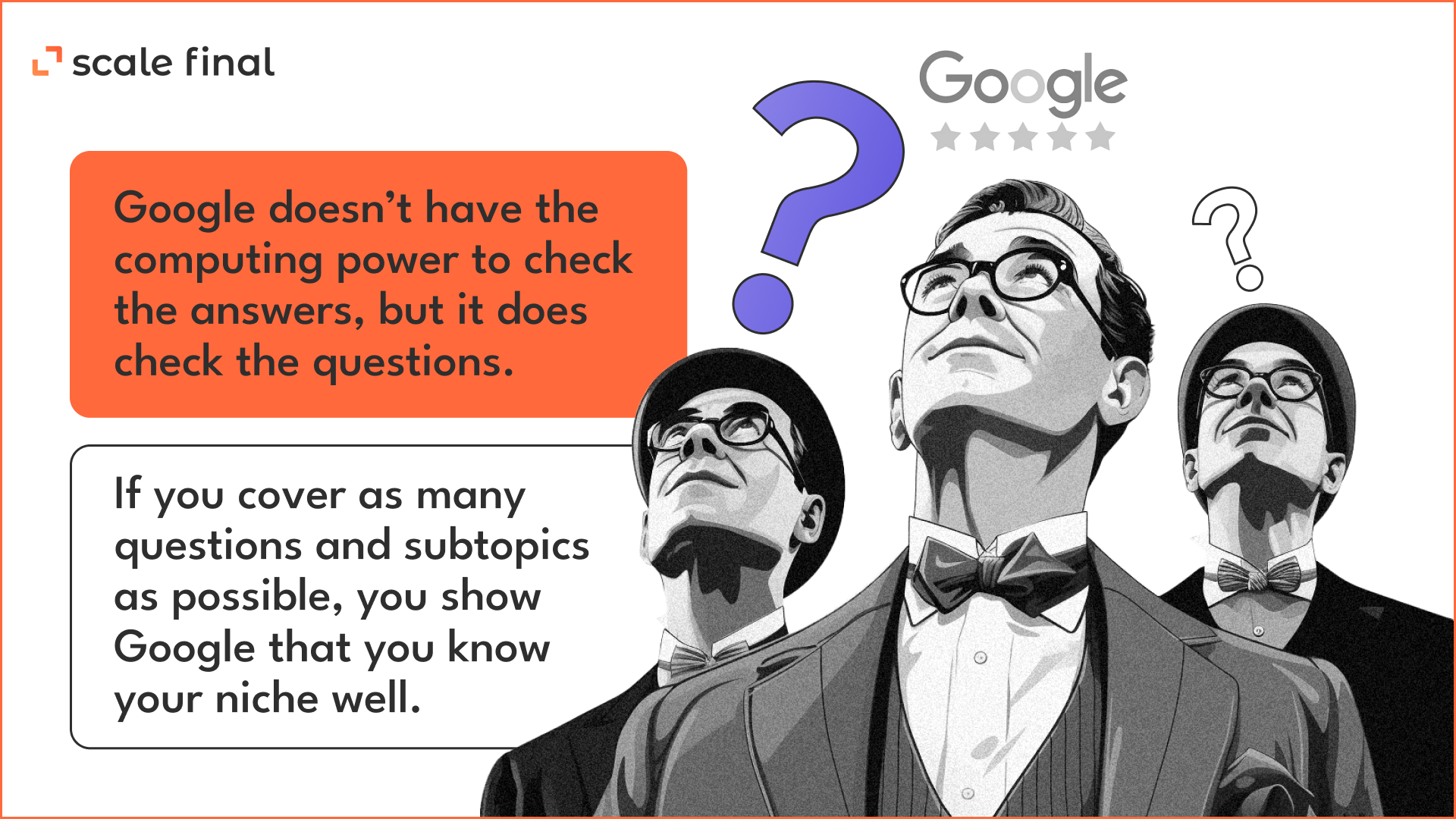
If you cover as many questions and subtopics as possible, you show Google that you know your niche well.
In 2021, another update, MUM, saw the light of day. Before it, Google’s used different AI models for different tasks, but with MUM, it’s just one model handling all tasks, allowing machine learning better performance.
The most relevant thing for SEO is that MUM isn’t just processing text for data mining but other media formats: images, audio and video, all contributing to the Knowledge Graph.
What this means for SEO
The last step in the evolution of Google has significant implications for SEO.
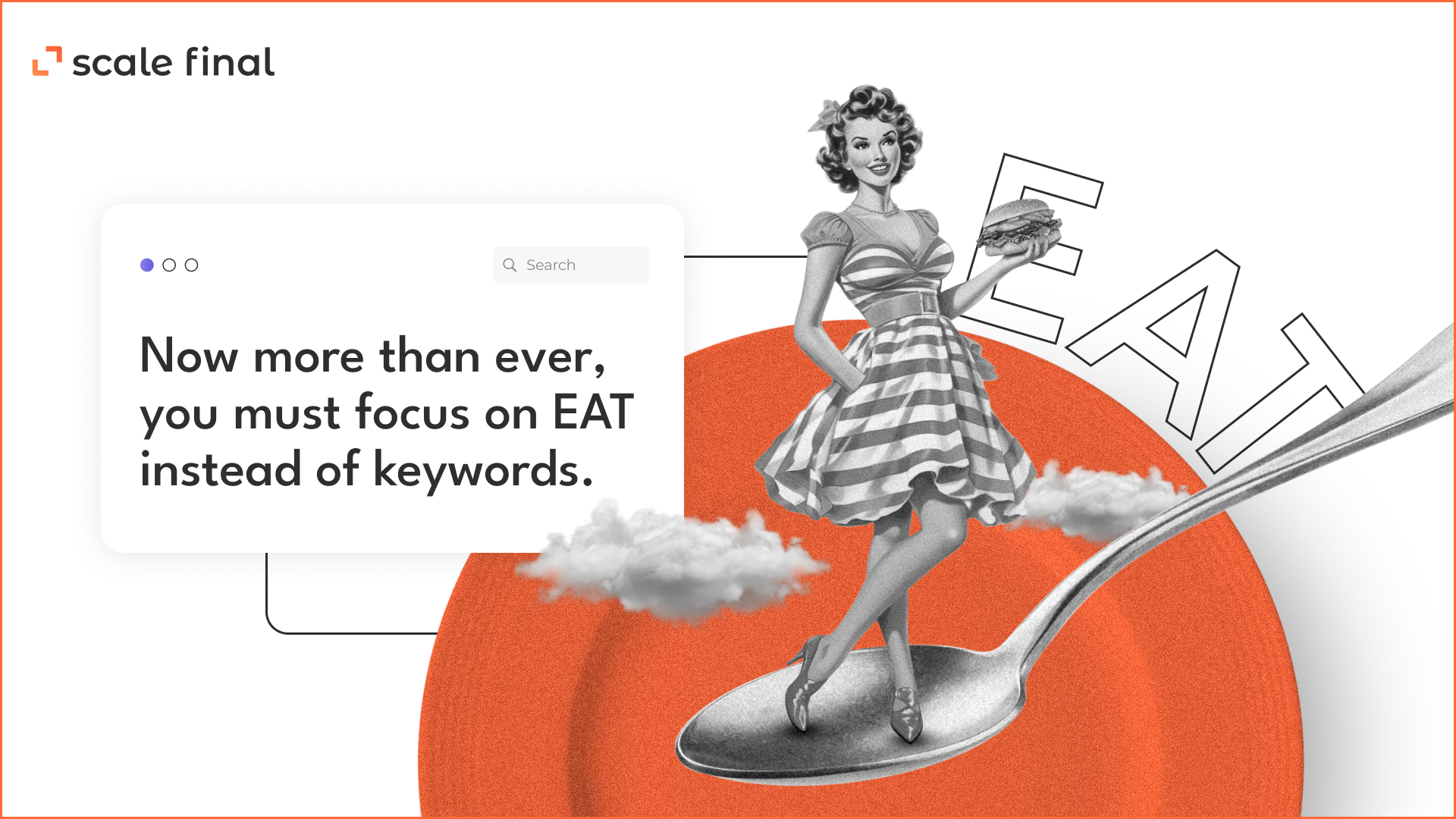
Google’s natural language processing needs less structured information. Technical SEO tasks, including markup with structured data, become inefficient.
Links, content, and other media formats are still relevant. By co-occurring in searches and content, links are signals of trust and authority.
The frequency of keywords is less important than the perspective from which a topic is approached. TF-IDF analysis is still how to find relevant terms regarding a topic’s keyword corpus.
When it comes to content, just creating it is not enough. Google is looking to assist users on their customer journey to receive traffic related to products and get a share of the market. Content marketing is key here.
Throughout users’ research on a topic, they may go from “What is content marketing” to “How does content marketing work” and then, realizing that there’s a lot to this topic, “Who provides content marketing services?”. And throughout this research process, companies need to have the answers for users.
Therefore, the content you create now must have the users and their questions and needs in mind. For this purpose, you should focus on SERP analytics.
Final words
It’s interesting to think about the Google evolution of this search engine. From manual analysis of statistically natural data points, to sophisticated machine learning, it’s come a long way.
Ultimately, no one knows precisely how Google’s ranking pages. It was simple in the old days, but with machine learning, Google interprets vast data points and makes decisions.
Still, at the point where it’s now, there are valuable SEO lessons to be extracted from how Google has developed MUM.
P.S. SEO is more than just understanding algorithms; it’s about anticipating change and adapting strategies for accurate results.
ScaleFinal is here to help and support you every step of the way.
Contact for a free SEO consultation and discover the difference expertise can make.
Author
-
CEO of ScaleFinal
I'm CEO of Scale Final and an experienced SEO specialist obsessed with trackable results and process optimization. My personal motto is "Get more by spending less". My interests and hobbies include: Yoga, tea culture, skateboarding, board games, Mafia social game, arthouse cinema, off-road cycling, psychology, Zen Buddhism and meditation.
View all posts